How To Build A Data Science Platform For Startups From Scratch
Today, data science has become a crucial product for startups to improve the product and performance. The various applications of data science for startups include the following.
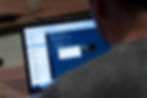
Discovering the important business metrics to measure as well as forecast
Constructing predictable prototypes of customer behavior
Conducting experiments for product changes testing
Developing data products that can facilitate new product characteristics
In fact, most organizations are struck up with the first two or three steps without benefiting from the complete potentials of the data science. Small teams can nevertheless make use of managed services to move ahead of data pipelines to perceive how data science can help in product development.
Step by step guide to deploying data science for product development
Data tracking
The entire process of deploying data science for product development starts with data capture from different applications and web pages. The users must first learn the different methods of gathering the tracking data. During the process, it is necessary to be familiar with the ideas of privacy and fraud.
Understanding data pipelines
The second step consists of working with the different approaches to data collection for analysis. It is necessary to know how to deal with flat files, data lakes, and database. Programs like PubSub, DataFlow, and BigQuery will be of immense help during this stage.
Data analysis
The most common data analysis used for delving deep into the data include constructing histograms and distribution functions. Correlation analysis follows then.
Predictive modeling
The analysts work with the different approaches to supervised and unsupervised learning and develop churn and cross-promotion predictive models. During this stage, analysts must also evaluate offline model performance.
Production of model
Offline models are scaled up to score a voluminous record. The business must now be familiar with batch and online approaches pertaining to model deployment.
Experimentation
This step involves A/B products testing and setting up an experimentation framework to run experiments. Following this, a recommender is scaled up for a production system.